Exciting Applications of Machine Learning in the Water Industry
Last Modified Jan 26, 2023
The water industry is beginning to recognize and apply machine learning (ML) as a tool to optimize system operations in a way that was not possible even a few years ago. This is primarily due to advances in online instrumentation, data management and Cloud computing.
At its simplest, ML is learning from data. Every day, various types of data are recorded on a massive scale throughout the water industry, and ML can be used to analyze these complex datasets, helping operators by leveraging the objective and powerful capabilities of computers to identify and utilize patterns from the data that a human may not recognize. Machine learning models are developed through model training, after which they are used to make predictions on “unseen data” – real-time data that will be brought into the model to provide knowledge or insights for decision-making. Well-trained (or calibrated) models can explore and process massive datasets in real time while also providing extremely rapid predictions, insights, and/or recommendations for operators—a difficult and sometimes impossible task for a human, especially in a short time frame.
Related Topics:
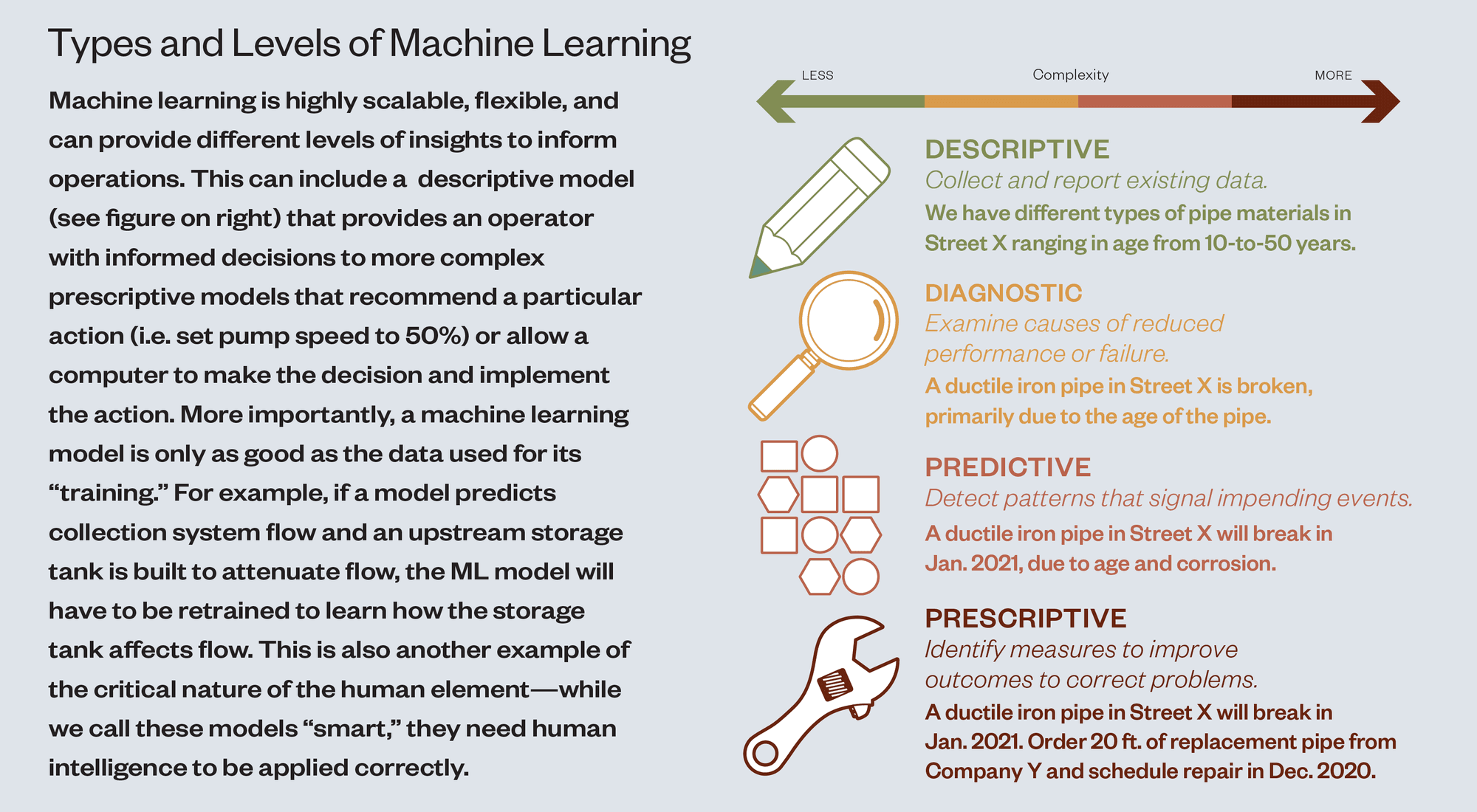
Machine learning is highly scalable, flexible, and can provide different levels of insights to inform operations.
That said, data-driven ML tools are meant to assist and not replace human intelligence, and they do not have to be complicated or involve massive amounts of data to be useful. Operational experience and expertise, rather, is fundamental to successful ML development, interpretation, and implementation. The use of water experts to develop an ML model is critical for integrating the science of water into the model. Once in production, it will always be important for a human to review the recommendations of the model, periodically verify the model is continuously learning, and apply their own judgment and experience to the question at hand.
One of the most compelling benefits of building ML models is that is allows the user to always have an up-to-date model of their system. This differs from most mechanistic modeling software packages that must be recalibrated by a human every couple of years and that likely have significant drift during that time period (e.g., biological process simulators, collection system models). In addition, ML models can account for some real-life complexity and nuances that may not be captured in mechanistic models (e.g., biological phosphorus modeling is often a simplified version of reality).
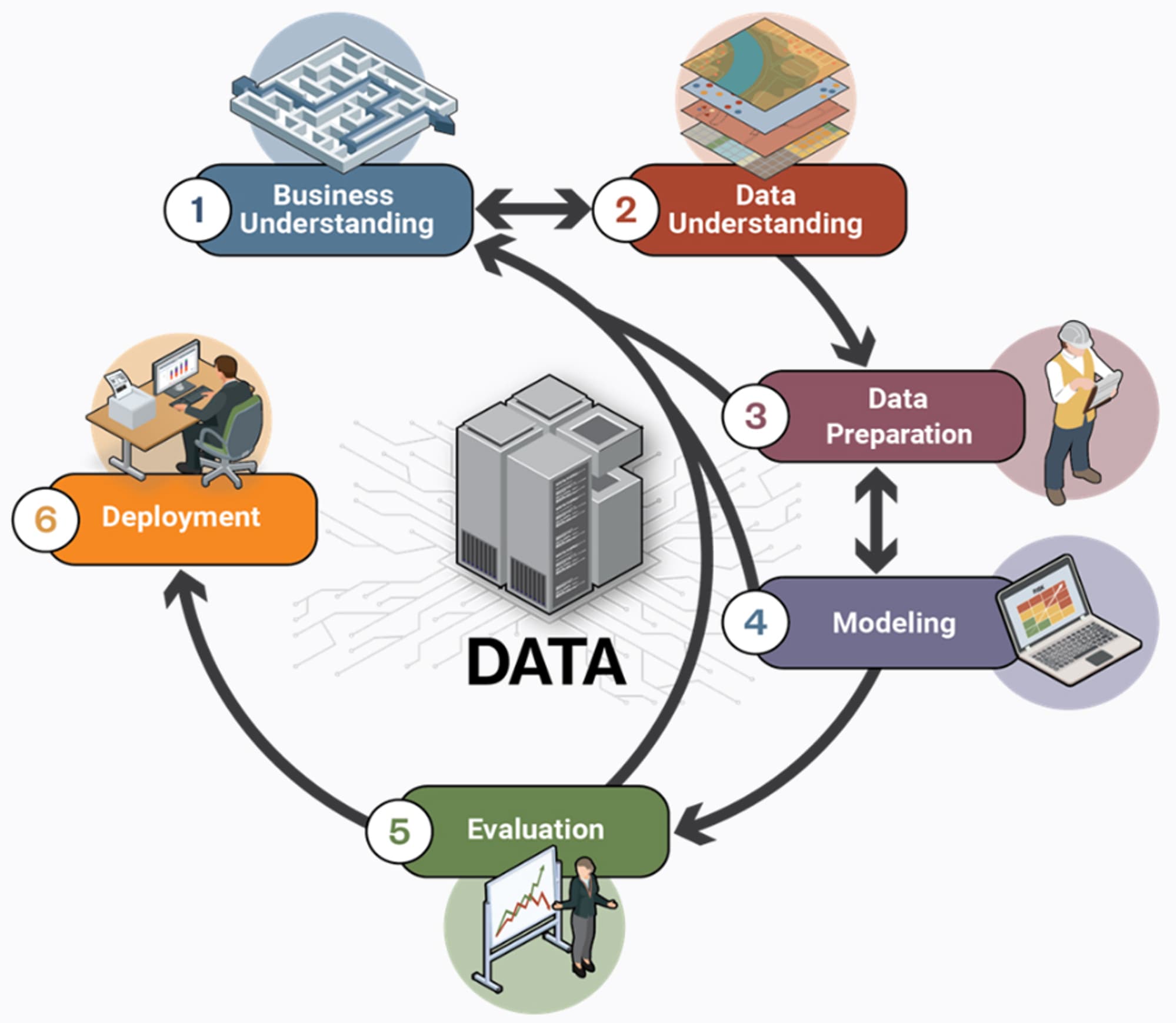
Two applications of ML in the water space—one a fully deployed model predicting influent wastewater flow for wet weather management, and the second a desktop model predicting the percent total solids (%TS) in cake on any given day:
Influent Flow Prediction Tool
In July 2020, Raleigh Water deployed an ML workflow that predicts influent flow to the Neuse River Resource Recovery Facility (NRRRF) 72-hours in advance. The tool is used to optimize the timing and flow trigger at which to use the equalization basin. The tool also provides the estimated peak hour flow that is used in the NRRRF’s secondary clarifier guidance program, which provides real-time state point analysis determining how many secondary clarifiers need to be online at a given flow, SVI, RAS flow, and mixed liquor concentration.
A well-calibrated model predicting influent flows to the NRRRF was developed using a ML-based approach. A ML model was trained to over six years of hourly influent flow data to predict influent flow using the following explanatory variables: the past 12 hours of influent flow, streamflow data, and rainfall data. The model utilizes hourly rainfall forecasts and real-time streamflow data in its predictive algorithm.
The predictions are displayed in a web-based Microsoft Power BI dashboard tool that includes the ability to estimate the optimal point to fill the equalization basin to maximize its utility. Microsoft Azure was used to develop an automated data pipeline to update model predictions each hour. Since deployment, there have been seven wet weather events, and three were major storm events including Hurricane Isaias.
The second and third major storm events were the only ones of the seven during which the NRRRF employed a significant portion of its equalization volume, utilizing 17 of the 32 MG available for the September storm and 27 MG of the 32 MG for the November storm. For the September event, the NRRRF was able to maintain excellent effluent quality as evidenced by the effluent values the day after the storm being similar to the day before. Note that the NRRRF only has to monitor effluent quality two times a week due to its historically superior performance, so daily samples were not available.
Cake %TS Predictor
We endeavored to determine if we could develop an empirical relationship between explanatory variables and dewaterability. The value propositions are that (1) enhanced understanding of the variables contributing to improved dewaterability allows the user to operate optimally to reduce hauling and disposal costs, and (2) polymer dose could be increased to assist on days with poor dewaterability.
Five years of data from a plant averaging 22% cake with an average polymer dose of 42 lb/DT was used for training. Seven explanatory variables including the influent carbon to nitrogen ratio times ash content (C/Nash) were used to predict %TS with a precision of +/- 0.4 %. Two separate models were generated to gain better insight into how the explanatory variables affect dewaterability. For example, both showed a positive correlation between increased C/Nash and %TS in cake as other studies have shown (CNash – A novel parameter predicting cake solids of dewatered digestates, O.K. Svennevik et al. / Water Research 158 (2019) 350-358). These models concluded that only 20 percent of the factors influencing dewatering are truly fixed properties, meaning the remainder are operational variables that could be modified to optimize dewaterability. Work is ongoing to better understand how these insights generated from ML compliment the ongoing mechanistic research in this area, and to see if deployment is of interest at any utilities.